AI breaks the ice in simulation of freezing H20
A team based at Princeton University has accurately simulated the initial steps of ice formation by applying artificial intelligence (AI) to solving equations that govern the quantum behavior of individual atoms and molecules.
The resulting simulation describes how water molecules transition into solid ice with quantum accuracy. This level of accuracy, once thought unreachable due to the amount of computing power it would require, became possible when the researchers incorporated deep neural networks, a form of artificial intelligence, into their methods.
The study was published in the journal Proceedings of the National Academy of Sciences (PNAS).
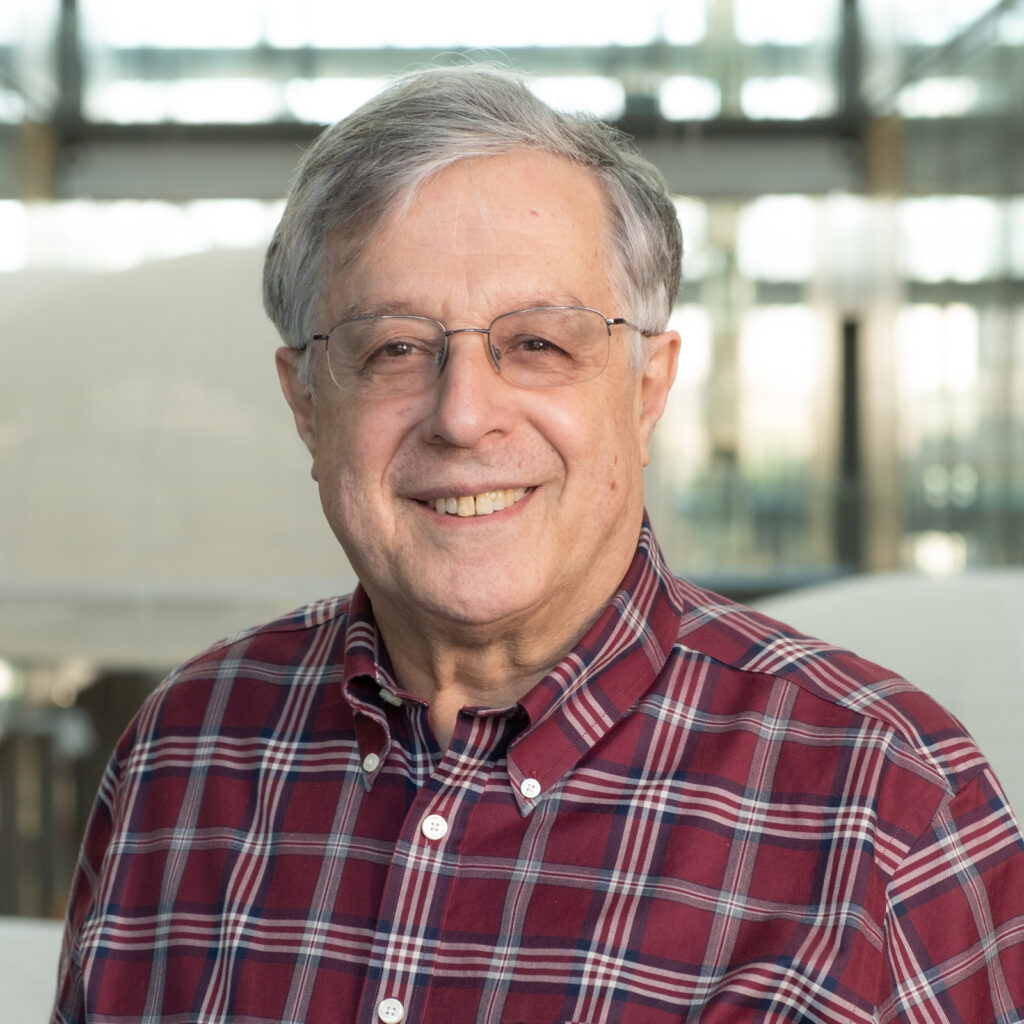
Roberto Car, Princeton’s Ralph W. *31 Dornte Professor in Chemistry.
“In a sense, this is like a dream come true,” said Roberto Car, Princeton’s Ralph W. *31 Dornte Professor in Chemistry, who co-pioneered the approach of simulating molecular behaviors based on the underlying quantum laws more than 35 years ago. “Our hope then was that eventually we would be able to study systems like this one, but it was not possible without further conceptual development, and that development came via a completely different field, that of artificial intelligence and data science.”
The ability to model the initial steps in freezing water, a process called ice nucleation, could improve accuracy of weather and climate modeling as well as other processing like flash-freezing food.
The new approach enables the researchers to track the activity of hundreds of thousands of atoms over time periods that are thousands of times longer, albeit still just fractions of a second, than in early studies.
Car co-invented the approach to using underlying quantum mechanical laws to predict the physical movements of atoms and molecules. Quantum mechanical laws dictate how atoms bind to each other to form molecules, and how molecules join with each other to form everyday objects.
Car and Michele Parrinello, a physicist now at the Istituto Italiano di Tecnologia in Italy, published their approach, known as ab initio (Latin for “from the beginning”) molecular dynamics, in a groundbreaking paper in 1985.
But quantum mechanical calculations are complex and take tremendous amounts of computing power. In the 1980’s, computers could simulate just a hundred atoms over spans of a few trillionths of a second. Subsequent advances in computing and the advent of modern supercomputers boosted the number of atoms and timespan of the simulation, but the result fell far short of the number of atoms needed to observe complex processes such as ice nucleation.
AI provided an attractive potential solution. Researchers train a neural network, named for its similarities to the workings of the human brain, to recognize a comparatively small number of selected quantum calculations. Once trained, the neural network can calculate the forces between atoms that it has never seen before with quantum mechanical accuracy. This “machine learning” approach is already in use in everyday applications such as voice recognition and self-driving automobiles.
Applying Deep Neural Networks
In the case of AI applied to molecular modeling, a major contribution came in 2018 when Princeton graduate student Linfeng Zhang, working with Car and Princeton professor of mathematics Weinan E, found a way to apply deep neural networks to modeling quantum-mechanical interatomic forces. Zhang, who earned his Ph.D. in 2020 and is now a research scientist at the Beijing Institute of Big Data Research, called the approach “deep potential molecular dynamics.”
In the current paper, Car and postdoctoral researcher Pablo Piaggi along with colleagues applied these techniques to the challenge of simulating ice nucleation. Using deep potential molecular dynamics, they were able to run simulations of up to 300,000 atoms using significantly less computing power, for much longer timespans than were previously possible. They carried out the simulations on Summit, one of the world’s fastest supercomputers, located at Oak Ridge National Laboratory.
This work provides one of the best studies of ice nucleation, said Pablo Debenedetti, Princeton’s dean for research and the Class of 1950 Professor of Engineering and Applied Science, and a co-author of the new study.
“Ice nucleation is one of the major unknown quantities in weather prediction models,” Debenedetti said. “This is a quite significant step forward because we see very good agreement with experiments. We’ve been able to simulate very large systems, which was previously unthinkable for quantum calculations.”
Currently, climate models obtain estimates of how fast ice nucleates primarily from observations made in laboratory experiments, but these correlations are descriptive, not predictive, and are valid over a limited range of experimental conditions. In contrast, molecular simulations of the type done in this study can produce simulations that are predictive of future situations, and can estimate ice formation under extreme conditions of temperature and pressure, such as on other planets.
“The fact that we are studying very complex phenomena from the fundamental laws of nature, to me that is very exciting,” said Piaggi, the study’s first author and a postdoctoral research associate in chemistry at Princeton.
“We start with the equation that describes how electrons behave. Electrons determine how atoms interact, how they form chemical bonds, and virtually the whole of chemistry.”
The atoms can exist in literally millions of different arrangements, said Car, who is director of the Chemistry in Solution and at Interfaces center, funded by the U.S. Department of Energy Office of Science and including regional universities.
“The magic is that because of some physical principles, the machine is able to extrapolate what happens in a relatively small number of configurations of a small collection of atoms to the countless arrangements of a much bigger system,” Car said.
Although AI approaches have been available for some years, researchers have been cautious about applying them to calculations of physical systems. In the last couple of years, however, there has been a significant change in this attitude, Piaggi said, not only because the algorithms work but also because researchers are using their knowledge of physics to inform the machine learning models.
For Car, it is satisfying to see the work started three decades ago come to fruition. “The development came via something that was developed in a different field, that of data science and applied mathematics,” Car said. “Having this kind of cross interaction between different fields is very important.”
This work was supported by the U.S. Department of Energy (grant DE-731 SC0019394) and used resources of the Oak Ridge Leadership Computing Facility (grant DE-AC05-00OR22725) at the Oak Ridge National Laboratory. Simulations were substantially performed using the Princeton Research Computing resources at Princeton University.
The study, “Homogeneous ice nucleation in an ab initio machine learning model of water,” by Pablo M. Piaggi, Jack Weis, Athanassios Z. Panagiotopoulos, Pablo G. Debenedetti, and Roberto Car, was published in the journal Proceedings of the National Academy of Sciences the week of August 8, 2022. DOI: /10.1073/pnas.2207294119.