Doyle Lab Reimagines Workflow For Substrate Scope Analysis
Revisiting the process through which chemists analyze substrate scopes, the Doyle Lab used data science to construct diverse and representative scopes for a concise, user-friendly tool for synthetic chemists. The tool broadly spans the chemical space, providing information about steric profiles, electronics, and compatibility with many common functional groups using a conserved number of molecules. It was developed during research that presents a versatile approach towards the alkylation of aryl halides using an alcohol-derived coupling partner.
PUBLICATION:
Journal of the American Chemical Society (JACS): “Using Data Science to Guide Aryl Bromide Substrate Scope Analysis in a Ni/Photoredox-Catalyzed Cross-Coupling with Acetals as Alcohol-Derived Radical Sources.”
AUTHORS:
Stavros Kariofillis, Shutian Jiang, Andrzej Żurański, Shivaani Gandhi, Jesus Martinez-Alvarado, Abigail Doyle.
WHY THEY TOOK ON THE PROJECT:
The research confronts several common criticisms of substrate scope tables: 1) limitations are often not reported because the publishing process rewards positive results and therefore low-yielding substrates are often not reported; and 2) these substrate scope tables may not be truly representative of the broader chemical space of the substrate class.
In addition, unless the exact molecule of interest falls into one of the substrate scope tables, it can be next to impossible to know how well the reaction will translate to a specific coupling partner, underscoring the importance of scopes that are maximally representative.
WHAT THEY DID:
Solving this challenge involved the integration of data science tools to analyze the chemical space of a substrate class (in this case, aryl bromides). Researchers started with a search of all substrates from a database, followed by filtering, featurization to extract features of each substrate relative to their physical organic properties, and then dimensionality reduction such that they could visualize the chemical space in two-dimensions with substrates plotted by similarity. This set of ~2700 aryl bromides was divided into clusters, and selection of the centermost molecule per cluster enabled them to generate a scope that is maximally covered and representative.
QUOTE FROM LEAD AUTHOR STAVROS KARIOFILLIS*:
“As synthetic chemists, our training has largely been in synthesis and mechanistic analysis. Even though exposure to data science is not a traditional part of synthetic training, there are so many ways that integrating these techniques and machine learning can amplify synthetic chemistry.
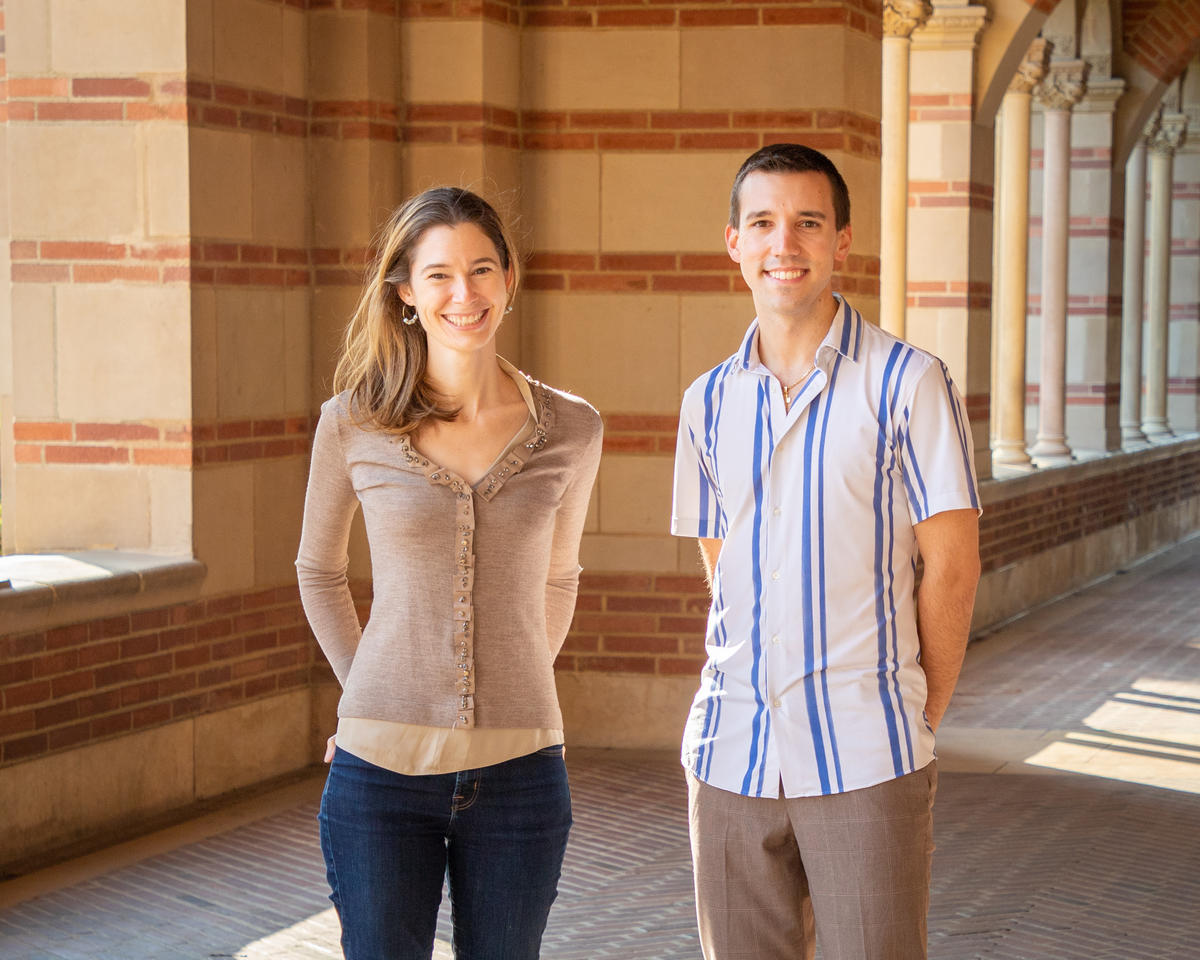
Photo courtesy of Stavros Kariofillis
For example, in developing and using this tool, we were able to extract a great deal of diversity from the molecules selected for evaluation in the substrate scope. This scope is comprised of a conserved number of molecules that maximally cover the aryl bromide chemical space. Included in this set of substrates were two 0% yields, which revealed information about the limits of steric bulk and electronics of the aryl bromide substrates. We report these 0% yields in the paper, and inclusion of these data points enabled us to build predictive models. Taken together, I hope these advances will give someone looking to translate this method to an unseen substrate advanced information on reaction performance.”
*Kariofillis successfully defended his dissertation this week to earn his doctoral degree.
QUOTE FROM P.I. ABIGAIL DOYLE:
“There has been a shift toward larger and larger scope tables within papers. Instead, Stavros and his co-workers pursued a general and quantitative scope selection workflow informed by studies in chemoinformatics and data science to select a maximally diverse and succinct collection of scope examples. The benefits of this approach are potentially multifold: it could standardize scope analysis and enable chemists to compare among methods that afford similar products; it could reduce the time and cost associated with scope evaluation; and it could afford literature better suited to quantitate modeling of reactivity in the long run.”
HOW TO ACCESS THE TOOL:
Researchers want users to be confident that they can follow this workflow even if they don’t have a background in data science. The annotated code is available in JACS’ supporting information, and autoQChem is free as a website link: (https://github.com/PrincetonUniversity/auto-qchem).
FUNDING:
This research is supported by funding from the National Science Foundation Graduate Research Fellowship Program (Grant Number DGE-1656466); the Schmidt DataX Fund at Princeton University, Schmidt Futures Foundation; the Princeton Innovation Fund, NIGMS (R35 GM126986) (Ni/photoredox method development); and the CCI Center for Computer Assisted Synthesis (CHE-1925607).
FULL PAPER:
https://pubs.acs.org/doi/10.1021/jacs.1c12203